AI-Powered Hiring: How Automation is Changing Recruitment Forever
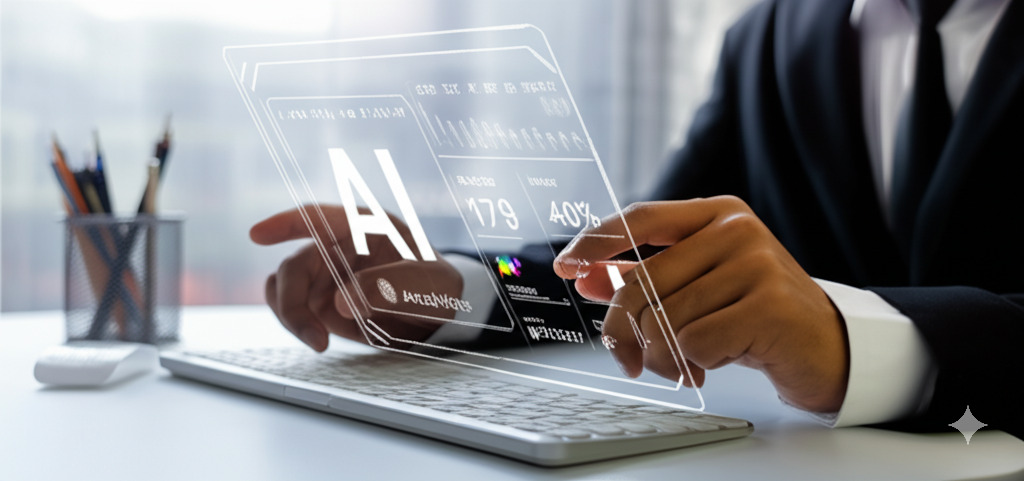
The world of work is in constant flux, but perhaps no function within business is undergoing as rapid and profound a transformation as recruitment. For decades, hiring has been a largely manual, time-intensive process, often fraught with inefficiencies, subjective biases, and mounting pressure to fill roles faster without compromising quality. Enter Artificial Intelligence (AI) and automation – technologies no longer confined to science fiction, but actively reshaping the talent acquisition landscape today. From sifting through thousands of resumes in seconds to predicting future workforce needs, AI-powered hiring is not just a trend; it’s a paradigm shift, promising unprecedented efficiency, data-driven decision-making, and potentially fairer outcomes. But how exactly is this technology revolutionizing hiring, what are the tangible benefits and inherent risks, and is the ‘human’ element of HR truly replaceable? This article delves into the rise of AI in recruitment, exploring its key applications, real-world impact, and the crucial considerations for organizations navigating this new era.
The AI Revolution in Talent Acquisition: More Than Just Buzzwords
Before diving into specific applications, it’s essential to understand what “AI” means in the context of hiring and why its adoption is accelerating. At its core, AI refers to computer systems capable of performing tasks that typically require human intelligence, such as learning, problem-solving, decision-making, and understanding language. In recruitment, this primarily involves:
- Machine Learning (ML): Algorithms that learn patterns and make predictions from data without being explicitly programmed. In hiring, ML powers resume analysis, candidate matching, and performance prediction.
- Natural Language Processing (NLP): The ability of computers to understand, interpret, and generate human language. NLP is crucial for resume parsing, chatbot interactions, and analyzing interview transcripts or open-ended survey responses.
- Predictive Analytics: Using historical and current data to forecast future outcomes. This is applied in workforce planning, identifying flight-risk employees, and predicting candidate success.
- Automation: While not strictly AI, automation often works hand-in-hand with it. Robotic Process Automation (RPA) can handle repetitive, rule-based tasks like scheduling interviews, sending standard communications, or moving candidates through ATS stages based on predefined triggers, freeing up human recruiters for more strategic work.
The surge in AI adoption is driven by several factors: the sheer volume of applications generated by online job postings, the need for speed in a competitive talent market, the desire for more objective, data-driven hiring decisions, and the increasing sophistication and accessibility of AI tools themselves. Companies are realizing that traditional methods struggle to keep pace, leading them to embrace technology that promises efficiency and smarter hiring.
AI in Resume Screening: From Keyword Matching to Contextual Understanding
One of the most time-consuming stages in recruitment is initial resume screening. Recruiters often face hundreds, if not thousands, of applications for a single opening. Manually reviewing each one is not only laborious but also prone to inconsistency and unconscious bias. This is where AI has made some of its earliest and most significant impacts.
Early Applicant Tracking Systems (ATS) relied heavily on basic keyword matching, often filtering out potentially great candidates whose resumes didn’t contain the exact terminology recruiters searched for. Modern AI-powered screening tools go far beyond this:
- Semantic Analysis (NLP): AI can understand the *meaning* and *context* behind the words on a resume, not just the words themselves. It recognizes related skills, understands nuances in job titles, and can infer capabilities even if they aren’t explicitly stated using specific keywords. For example, it might understand that “managed a P&L” implies financial responsibility, even if the exact phrase “budget management” isn’t present.
- Skill Extraction and Matching: AI algorithms can automatically extract key skills, experiences, and qualifications from diverse resume formats and structures. They can then score and rank candidates based on their alignment with the job requirements, considering factors like years of experience, proficiency levels, and the relevance of past roles.
- Predictive Performance Indicators: Some advanced tools use ML trained on historical hiring data (performance reviews, tenure of past hires) to identify patterns in resumes that correlate with future job success *within that specific company or role*. This attempts to move beyond just matching qualifications to predicting potential.
- Bias Mitigation Features: Conscious of the potential for bias, many AI screening tools offer features to anonymize resumes by removing names, photos, graduation years, and other demographic indicators during the initial review, forcing the evaluation to focus solely on skills and experience.
Benefits: The advantages are clear – drastic reduction in time spent on screening, increased consistency in evaluation (applying the same criteria to all applicants), the ability to consider a larger talent pool, and, when implemented carefully, the potential to reduce human bias. Recruiters can focus their energy on engaging with the most qualified short-listed candidates.
Caveats: The primary concern is algorithmic bias. If the AI is trained on historical data reflecting past biased hiring practices, it can perpetuate or even amplify those biases. Continuous monitoring, auditing, and using diverse training datasets are crucial.
Automating and Enhancing Interviews: Efficiency Meets Insight?
AI’s influence extends beyond screening into the interview process itself, streamlining logistics and aiming to provide deeper insights:
- Recruitment Chatbots: AI-powered chatbots can engage candidates 24/7 on career sites or messaging platforms. They answer frequently asked questions, provide company information, conduct initial pre-screening (asking basic qualifying questions), and even schedule interviews, improving candidate engagement and freeing up recruiter time.
- Automated Interview Scheduling: Coordinating availability between candidates and multiple interviewers is a common headache. AI tools can integrate with calendars, find mutually convenient times, send invites, and handle rescheduling automatically.
- Video Interview Analysis: This is perhaps the most discussed and controversial application. Candidates record video responses to standardized questions. AI platforms then analyze various aspects:
- Transcript Analysis (NLP): Analyzing the content of the candidate’s answers for relevant keywords, skills, and alignment with job requirements.
- Speech Pattern Analysis: Analyzing tone of voice, pace of speech, and filler word usage.
- Facial Expression Analysis (Less Common/More Controversial): Some tools claim to analyze micro-expressions to infer personality traits or emotional states. This area faces significant scientific skepticism and ethical concerns regarding accuracy and potential for bias.
- AI-Powered Assessment Platforms: Beyond traditional interviews, AI is used in skills assessments (e.g., coding challenges with AI-driven scoring and feedback) and gamified assessments that evaluate cognitive abilities or personality traits in engaging ways.
Benefits: Increased efficiency in scheduling and initial screening, standardized interview questions for better comparability, potential for insights beyond the resume (especially from content analysis), and flexibility for candidates (e.g., recording video interviews at their convenience).
Challenges: Candidate acceptance and comfort with automated or analyzed interviews can vary. The accuracy and validity of AI analysis, particularly regarding personality or sentiment from video/audio, are heavily debated and require careful validation. Ensuring fairness and avoiding biases encoded into these analytical models is paramount. Over-reliance on such tools without human judgment can lead to poor decisions and negative candidate experiences.
Predictive Power: AI in Strategic Workforce Planning
AI’s role extends beyond filling immediate vacancies to shaping long-term talent strategy. By analyzing vast datasets, AI can provide insights crucial for strategic workforce planning:
- Forecasting Hiring Needs: Analyzing historical hiring trends, business growth projections, market dynamics, and employee turnover data, AI models can predict future staffing requirements across different departments and roles with greater accuracy than traditional methods. This allows TA teams to proactively build talent pipelines for anticipated needs.
- Identifying Skill Gaps: AI can analyze the current workforce’s skill inventory (from performance data, project involvement, profiles) and compare it against future business strategy requirements or emerging industry trends. This highlights potential skill gaps that need to be addressed through hiring, upskilling, or reskilling.
- Predicting Employee Turnover: By analyzing factors like tenure, compensation, performance reviews, promotion history, commute times, and even sentiment from employee surveys, AI models can identify employees or groups at higher risk of leaving. This enables proactive retention efforts targeted at key talent.
- Optimizing Job Descriptions: AI tools can analyze the performance of past job postings (e.g., applicant volume, quality, diversity) and suggest improvements to wording, required qualifications, or even the title to attract a broader or more suitable pool of candidates. Some tools can also scan descriptions for potentially biased language.
- Succession Planning: AI can help identify high-potential internal candidates for future leadership roles by analyzing performance data, skill sets, and career progression patterns, integrating internal mobility more effectively with talent needs.
Benefits: This shifts recruitment from a reactive function to a strategic, forward-looking one. It enables better resource allocation, reduces time-to-fill for future roles through proactive pipelining, aligns talent strategy more closely with business goals, and can improve retention of critical employees.
Enhancing the Candidate Experience Through Personalization and Efficiency
While fears of depersonalization exist, AI can paradoxically be used to create a *more* personalized and positive candidate experience when implemented thoughtfully:
- Personalized Job Recommendations: AI algorithms on career sites can analyze a candidate’s profile, resume, or browsing history to suggest roles that best match their skills and interests, similar to recommendation engines on consumer websites.
- Intelligent Chatbot Support: As mentioned, chatbots provide instant answers to common questions, guiding candidates through the application process and reducing frustration caused by lack of information or slow response times.
- Faster Feedback Loops: Automation speeds up processes like screening and scheduling, allowing recruiters to provide feedback (even if automated rejection notices) more quickly, which candidates consistently value.
- Tailored Communications: AI-powered CRM (Candidate Relationship Management) systems can help segment talent pools and send more relevant, personalized communications (e.g., company news, industry insights, relevant job alerts) to nurture passive candidates effectively.
The key is using AI to handle high-volume, low-touch interactions efficiently, freeing up human recruiters to provide high-touch, personalized engagement where it matters most – with qualified, interested candidates during later interview stages and offer negotiations.
Case Studies: AI Delivering Results in Recruitment
While specific vendor names and detailed metrics are often proprietary, the impact of AI in hiring is evident across industries. Here are illustrative examples based on common implementations:
- Case Study 1: High-Volume Retailer (“GlobalMart”)
- Challenge: Hiring thousands of seasonal and frontline staff across numerous locations quickly, overwhelming local managers with applications. High screening time, inconsistent candidate experience.
- AI Solution: Implemented an AI-powered ATS with advanced resume parsing and matching, coupled with chatbots for initial screening questions (eligibility, availability) and automated interview scheduling with store managers.
- Reported Results:
- Reduced time-to-hire by 40% for frontline roles.
- Screening time per applicant reduced from minutes to seconds.
- Improved candidate satisfaction scores due to faster responses and easier scheduling.
- Freed up store managers’ time to focus on final interviews and onboarding.
- Case Study 2: Mid-Sized Tech Company (“Innovate Solutions”)
- Challenge: Difficulty sourcing and evaluating highly specialized software engineers. Recruiters struggled to accurately assess niche technical skills from resumes alone, leading to wasted interview time with unqualified candidates.
- AI Solution: Adopted an AI sourcing tool to identify passive candidates on platforms like GitHub and Stack Overflow based on code repositories and technical contributions. Integrated an AI-powered coding assessment platform into the early screening process. Used NLP to better match resume skills to complex job requirements.
- Reported Results:
- Improved quality of candidates reaching the technical interview stage by 30%.
- Reduced recruiter time spent on unqualified technical screenings.
- Expanded reach into passive candidate pools with relevant niche skills.
- Better prediction of on-the-job technical performance based on assessment scores.
- Case Study 3: Financial Services Firm (“SecureInvest”)
- Challenge: Concerns about unconscious bias impacting diversity in hiring funnels, particularly at the initial screening stage. Desire for a more objective and equitable process.
- AI Solution: Implemented an AI screening tool with robust anonymization features (hiding demographic data) and bias detection capabilities (flagging potentially biased language in job descriptions). Used AI analytics to monitor diversity metrics at each stage of the hiring process.
- Reported Results:
- Increased representation of underrepresented groups in interview shortlists by 15% within the first year.
- Identified and removed biased language from standard job templates.
- Provided data-driven evidence of a fairer initial screening process, improving employer brand perception.
- Required ongoing audits to ensure the AI itself wasn’t introducing new biases.
Navigating the Labyrinth: Challenges and Ethical Considerations
Despite the immense potential, the adoption of AI in hiring is not without significant challenges and ethical hurdles that must be carefully managed:
- Algorithmic Bias: This remains the most critical concern. AI systems learn from data, and if that data reflects historical societal or organizational biases (e.g., hiring more men for technical roles), the AI can learn and perpetuate these biases, potentially leading to discriminatory outcomes at scale. Mitigation requires careful algorithm design, diverse and representative training data, regular bias audits, transparency in how decisions are supported by AI, and robust human oversight.
- Data Privacy and Security: Hiring processes handle vast amounts of sensitive personal data. Organizations must ensure their AI tools and processes comply with regulations like GDPR, CCPA, and others. Transparency with candidates about how their data is being used and processed by AI systems is crucial for building trust.
- The “Human Touch” Dilemma: Over-reliance on automation can lead to a depersonalized and cold candidate experience. Candidates may feel alienated if they only interact with bots or feel unfairly judged by an algorithm. Balancing efficiency with meaningful human interaction, especially during later stages, feedback delivery, and relationship building, is essential. AI should augment, not entirely replace, human judgment and empathy.
- Accuracy, Validity, and Transparency: How accurate are the predictions or analyses made by AI tools, especially those claiming to assess personality or potential? The “black box” nature of some complex algorithms makes it hard to understand *why* a certain recommendation was made, hindering accountability and trust. Vendors need to be transparent about their methodologies, and organizations need to validate the tools’ effectiveness in their specific context.
- Implementation Costs and Change Management: Implementing sophisticated AI tools requires financial investment, technical expertise, and significant change management within the TA team and the broader organization. Recruiters need training to use these tools effectively and interpret their outputs correctly.
- Candidate Acceptance and Perception: Candidates’ willingness to engage with AI-driven processes varies. Concerns about fairness, privacy, and lack of human connection can lead some candidates to disengage. Clear communication about the use of AI and its benefits (like faster processing) can help alleviate some concerns.
The Future of AI in Recruitment: Towards Hyper-Personalization and Strategic Partnership
The evolution of AI in recruitment is far from over. Future trends likely include:
- Hyper-Personalization: AI enabling even more tailored candidate journeys, communications, and job recommendations based on deeper analysis of individual profiles and preferences.
- Sophisticated Predictive Models: More accurate predictions for candidate success, retention, and internal mobility, incorporating a wider range of data points.
- Seamless Integration: Tighter integration between external recruitment AI tools and internal HR systems (HRIS, Learning Management Systems) to provide a holistic view of talent from acquisition through the entire employee lifecycle.
- Enhanced Bias Detection and Mitigation: Continued focus on developing AI that is not only efficient but also demonstrably fair and equitable, with built-in auditing and explainability features.
- AI as a Strategic Advisor: AI tools providing TA leaders with high-level insights and recommendations on talent strategy, market intelligence, and process optimization, acting more like a partner than just an automation tool.
- Evolving Role of Recruiters: As AI handles more administrative and screening tasks, recruiters will focus increasingly on strategic sourcing, employer branding, candidate engagement, complex negotiations, and relationship management – skills where human intelligence and empathy remain paramount.
Conclusion: Embracing the Future Responsibly
AI and automation are undeniably changing recruitment forever. They offer powerful solutions to long-standing challenges, promising greater efficiency, data-driven insights, enhanced candidate experiences, and more strategic workforce planning. The potential benefits – faster hiring, better quality matches, reduced bias (when implemented correctly), and freeing up human recruiters for higher-value tasks – are compelling.
However, this transformation is not automatic or without risks. The ethical considerations, particularly around bias and privacy, are significant and demand careful attention. The fear of losing the human element is valid if technology is implemented carelessly. Success lies not in simply adopting AI, but in adopting it *responsibly*. This means choosing the right tools, rigorously validating their fairness and accuracy, ensuring transparency, investing in training, maintaining human oversight, and strategically integrating AI to augment, not replace, the essential human skills of empathy, judgment, and relationship building.
The future of recruitment isn’t about humans versus machines; it’s about humans *empowered* by machines. Organizations that successfully navigate this transition, leveraging AI ethically and effectively while keeping the candidate experience central, will gain a significant competitive advantage in the ongoing war for talent. The revolution is here, and adapting thoughtfully is no longer optional.