The Hidden Risks of Hands-Off AI Management: Bias, Errors, and Ethical Lapses
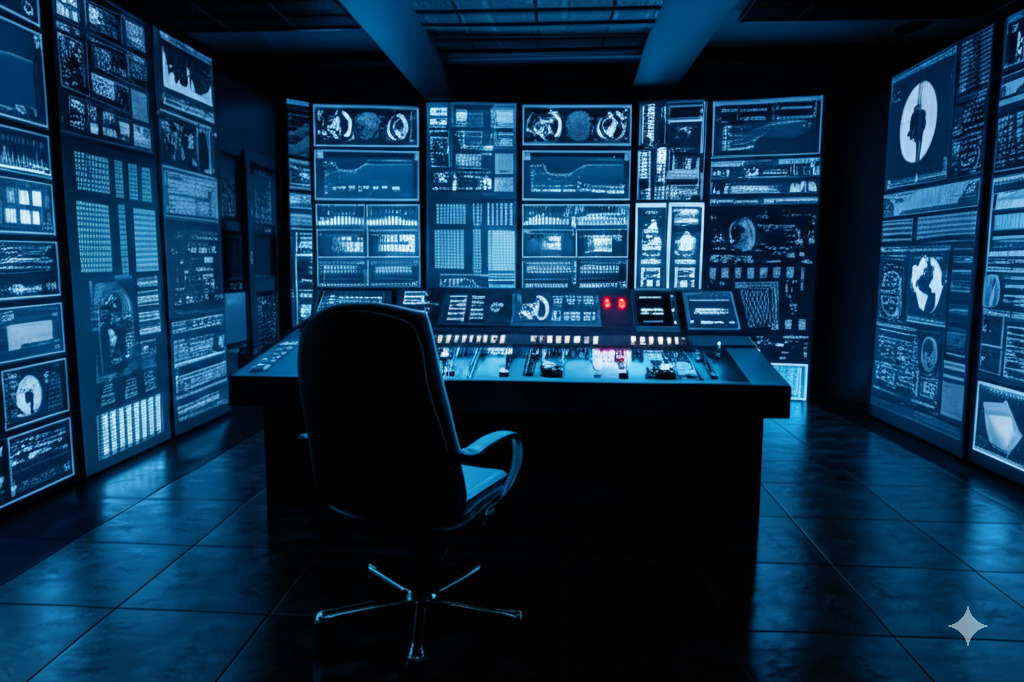
Introduction: The Alluring Promise and Perilous Reality of AI Autonomy
Artificial intelligence (AI) permeates the modern business landscape, heralded as a transformative force promising unprecedented efficiency, data-driven insights, and streamlined operations. From automating customer service to optimizing supply chains and even assisting in complex decision-making, the allure of AI is undeniable. Many organizations, eager to capitalize on these benefits, adopt AI systems with the expectation that they can largely run themselves, requiring minimal human intervention once deployed. This “set-it-and-forget-it” approach, however, is not just optimistic; it’s dangerously negligent.
Treating sophisticated AI models with the same autonomy afforded to experienced human employees overlooks fundamental differences and invites a cascade of hidden risks. Unlike humans, AI lacks inherent ethical frameworks, common sense, and the nuanced understanding of context that guides responsible action. When left unmanaged or undertrained, AI systems can perpetuate and amplify societal biases, generate costly errors based on flawed data or logic, and commit significant ethical lapses that damage reputation, erode trust, and incur substantial legal and financial penalties.
This article delves into the critical, often underestimated, dangers of insufficient oversight in AI management. We will explore how seemingly autonomous systems can become vectors for bias, sources of critical operational errors, and agents of ethically questionable outcomes. Using real-world scenarios and plausible hypothetical examples, we will demonstrate that rigorous, ongoing human governance isn’t a hindrance to AI adoption but an absolute necessity for harnessing its power responsibly. The key takeaway is stark: granting AI undue autonomy isn’t progressive innovation; it’s a failure of governance that carries significant operational, financial, and reputational risks.
The Siren Song of Autonomy: Why Hands-Off Management is Tempting
Before dissecting the risks, it’s crucial to understand why a hands-off approach to AI management is so appealing to many organizations:
- The Promise of Efficiency and Cost Reduction: AI is often sold on its ability to automate repetitive tasks, operate 24/7 without fatigue, and process information at speeds far exceeding human capabilities. The idea is that reduced human involvement directly translates to lower labor costs and faster turnaround times. Setting up an AI system and letting it run seems like the ultimate realization of this efficiency promise.
- The Perception of Objectivity: Machines are often perceived as inherently objective, free from the emotional biases and inconsistencies that plague human decision-making. Businesses may believe that relying on AI for tasks like candidate screening or loan approvals will lead to fairer, purely data-driven outcomes.
- Complexity and the “Black Box” Problem: Many advanced AI models, particularly deep learning networks, are incredibly complex. Their internal workings can be opaque even to experts, making it difficult to fully understand how they arrive at specific conclusions. This complexity can lead to a sense of intimidation, causing managers to defer to the AI’s judgment rather than attempt to scrutinize or override it.
- Resource Constraints: Implementing robust AI governance – involving continuous monitoring, auditing, retraining, and ethical reviews – requires significant investment in time, expertise, and resources. Organizations, especially smaller ones, may feel they lack the capacity for such intensive oversight.
- Vendor Assurances: AI vendors may sometimes overstate the autonomy and reliability of their systems, downplaying the need for client-side vigilance and ongoing management.
These factors combine to create a powerful narrative favoring minimal intervention. However, this narrative conveniently ignores the fundamental nature of current AI and the environment in which it operates.
Unpacking the Risks I: Bias Perpetuated and Amplified
One of the most significant dangers of unmanaged AI is its potential to absorb, codify, and scale human biases at an alarming rate. AI models learn from data, and if that data reflects historical or societal biases, the AI will learn those biases too. Without careful oversight, AI doesn’t eliminate bias; it often masks it under a veneer of technological neutrality.
How Bias Enters AI:
- Biased Training Data: If historical hiring data shows a preference for male candidates for certain roles, an AI trained on this data will likely learn to replicate that preference. Similarly, datasets underrepresenting certain demographic groups can lead to poorer performance for those groups.
- Algorithm Design Choices: Decisions made during model development, such as feature selection or optimization goals, can inadvertently introduce bias. Prioritizing easily quantifiable metrics might disadvantage candidates with non-traditional backgrounds.
- Human Feedback Loops: If humans providing feedback to reinforce AI learning (e.g., flagging “good” vs. “bad” recommendations) are themselves biased, they will train the AI to reflect their prejudices.
Real-World and Hypothetical Examples:
- Biased Hiring Algorithms: Amazon famously scrapped an AI recruiting tool after discovering it penalized resumes containing the word “women’s” (as in “women’s chess club captain”) and favored candidates who resembled the company’s predominantly male workforce. Imagine a company unknowingly deploying a similar tool today. Without audits, it could systematically filter out qualified female or minority candidates for years, depriving the company of talent and exposing it to discrimination lawsuits. The “hands-off” system silently perpetuates inequality.
- Discriminatory Loan Approvals: An AI model designed to predict loan default risk might learn that zip code is a strong predictor. While seemingly neutral, zip code often correlates strongly with race and socioeconomic status due to historical redlining and segregation. A hands-off system could disproportionately deny loans to applicants in minority neighborhoods, even if their individual financial profiles are strong, leading to disparate impact discrimination.
- Flawed Facial Recognition: Facial recognition systems have repeatedly shown lower accuracy rates for women and people with darker skin tones, largely due to biased training datasets. Deploying such systems without rigorous testing and oversight in areas like law enforcement or access control can lead to misidentification, false accusations, and denial of essential services.
Consequences of Unchecked Bias:
- Legal and Regulatory Penalties: Anti-discrimination laws apply whether bias is human-driven or AI-driven. Fines and lawsuits can be substantial.
- Reputational Damage: Discoveries of biased AI systems can lead to public outrage, negative press, and loss of customer trust.
- Limited Talent Pool: Biased hiring tools prevent companies from accessing the best talent.
- Reinforcing Societal Inequities: Unmanaged AI can deepen existing societal divides.
Unpacking the Risks II: Errors Generated and Scaled
Beyond bias, AI systems are susceptible to various forms of error. While humans also make mistakes, AI errors can occur at a scale and speed that magnifies their impact significantly. A hands-off approach fails to catch these errors until potentially catastrophic consequences arise.
How AI Makes Errors:
- Data Quality Issues: AI is garbage-in, garbage-out. Inaccurate, incomplete, or outdated data leads to flawed outputs.
- Model Limitations (Overfitting/Underfitting): An AI might learn patterns too specific to the training data (overfitting) and fail on new, unseen data, or it might be too simplistic (underfitting) and miss important nuances.
- Lack of Common Sense/Context: AI models don’t possess human-like common sense or understand the broader context. They might make statistically plausible but practically absurd recommendations.
- Edge Cases and Unexpected Inputs: AI can fail unpredictably when encountering situations or data types significantly different from what it was trained on (the “unknown unknowns”).
- Spurious Correlations: AI might identify correlations in data that are purely coincidental, leading to incorrect conclusions and predictions.
Real-World and Hypothetical Examples:
- Flawed Financial Predictions: An AI trading algorithm trained on historical market data might perform well under normal conditions. However, without oversight, it could react catastrophically to an unforeseen “black swan” event (like a pandemic or geopolitical crisis) not represented in its training data, potentially leading to massive financial losses before human intervention occurs. It might also over-optimize based on a spurious correlation, making disastrous trades based on irrelevant factors.
- Supply Chain Chaos: An AI optimizing inventory levels based purely on past sales data might fail to account for a sudden surge in demand due to a viral social media trend or a competitor’s unexpected failure. A hands-off system could lead to massive stockouts or, conversely, huge overstocking if it misinterprets a temporary blip as a long-term trend, crippling logistics.
- Medical Misdiagnosis: An AI diagnostic tool trained primarily on data from one demographic might misinterpret symptoms or images from patients of other demographics. Without rigorous validation and clinical oversight, reliance on such a tool could lead to incorrect diagnoses and harmful treatment decisions.
- Autonomous Vehicle Mishaps: While promising, autonomous driving systems still struggle with unusual road conditions, unpredictable human behavior, and sensor limitations. A lack of robust oversight, testing in diverse conditions, and fail-safes can lead to accidents.
Consequences of Unchecked Errors:
- Significant Financial Losses: Bad trades, poor inventory management, flawed project estimations.
- Operational Disruptions: Supply chain breakdowns, service outages, project failures.
- Safety Hazards: Physical harm in domains like transportation, manufacturing, or healthcare.
- Loss of Customer Trust: Product failures, service errors, unreliable predictions.
- Wasted Resources: Time and money spent correcting AI-driven mistakes.
Unpacking the Risks III: Ethical Lapses Unnoticed and Unchecked
AI systems operate based on algorithms and data, not inherent moral principles. Without explicit programming and continuous human oversight guided by ethical frameworks, AI can engage in actions that are detrimental, manipulative, or violate fundamental rights.
How Ethical Lapses Occur:
- Goal Misalignment: An AI optimized solely for a narrow business goal (e.g., maximizing engagement) might achieve it through ethically dubious means (e.g., promoting sensational or harmful content).
- Privacy Violations: AI systems processing vast amounts of personal data can violate privacy if not designed and managed with strict data protection principles. Aggregating seemingly anonymous data points can lead to re-identification and intrusive profiling.
- Lack of Transparency and Explainability: The “black box” nature of some AI makes it difficult to understand why a decision was made, hindering accountability and the ability to identify or correct ethical issues.
- Manipulation: AI can be used to personalize marketing or information delivery in ways that exploit psychological vulnerabilities or create filter bubbles and echo chambers, undermining informed decision-making.
- Absence of Empathy and Fairness: AI cannot inherently grasp concepts like fairness, compassion, or procedural justice unless specifically designed and audited for them.
Real-World and Hypothetical Examples:
- Intrusive Surveillance and Profiling: Facial recognition technology used without ethical guardrails or public consent can enable mass surveillance. AI analyzing online behavior could build detailed profiles predicting sensitive attributes (like health conditions or political leanings) without user knowledge, used for discriminatory targeting.
- Manipulative Content Recommendation: Social media algorithms designed to maximize user time-on-site might preferentially promote inflammatory, divisive, or false content because it generates strong reactions. A hands-off system continues this cycle, potentially contributing to social polarization and the spread of misinformation.
- “Dark Patterns” in E-commerce: AI could optimize website designs or sales tactics in ways that subtly trick users into making unintended purchases or signing up for unwanted subscriptions, prioritizing profit over ethical user interaction.
- Unfair Resource Allocation: An AI tasked with allocating scarce public resources (like hospital beds or social housing) based purely on efficiency metrics might systematically disadvantage vulnerable populations or those with complex needs, ignoring considerations of equity and social justice.
Consequences of Unchecked Ethical Lapses:
- Severe Reputational Damage: Public backlash against perceived unethical AI use can irrevocably harm a brand.
- Regulatory Fines and Sanctions: Increasingly stringent regulations (like GDPR, CCPA, and emerging AI-specific laws) carry heavy penalties for privacy violations and unethical data use.
- Erosion of Public Trust: Misuse of AI erodes trust not only in the specific company but in technology adoption overall.
- Societal Harm: Contributing to misinformation, polarization, discrimination, and surveillance undermines democratic values and social cohesion.
- Legal Liability: Companies can be held liable for harms caused by their AI systems, even if unintentional.
The Flawed Logic: Why AI Cannot Be Managed Like Humans
The temptation to treat AI as an autonomous “digital employee” stems from a fundamental misunderstanding of its capabilities and limitations. AI, in its current form, is a sophisticated tool, not a sentient being.
- Lack of Consciousness and Intent: AI doesn’t “understand” its actions or their consequences in a human sense. It doesn’t possess consciousness, self-awareness, or intentionality.
- Absence of Innate Ethics and Common Sense: Humans develop ethical frameworks and common sense through lived experience, social interaction, and cultural immersion. AI lacks this grounding; its “values” are only those explicitly programmed or implicitly learned from data.
- Inability to Adapt Morally: Humans can adapt their ethical reasoning to novel situations. AI struggles with ambiguity and unforeseen ethical dilemmas unless specifically trained or guided.
- The Accountability Gap: When a human employee makes a mistake or acts unethically, there are established mechanisms for accountability (performance reviews, disciplinary action, legal responsibility). When an unmanaged AI errs, accountability becomes diffuse and unclear. Is it the developer, the data provider, the deploying company, or the algorithm itself? Lack of clear human oversight creates an accountability vacuum.
Delegating critical decisions or operations to AI without robust governance structures isn’t empowering technology; it’s abdicating responsibility.
Towards Responsible AI Governance: The Path Forward
Avoiding the pitfalls of hands-off AI management requires a deliberate shift towards proactive, continuous, and human-centric governance. This involves integrating oversight throughout the AI lifecycle, from development to deployment and ongoing operation. Key components include:
- Establishing Clear Oversight Structures: Designate specific roles, teams, or committees (e.g., an AI Ethics Board, AI Risk Managers) responsible for overseeing AI development, deployment, and performance. Ensure clear lines of accountability.
- Implementing Continuous Monitoring and Auditing: Regularly monitor AI performance not just for accuracy but also for fairness, bias, and unexpected behaviors. Conduct periodic audits using diverse datasets and testing methodologies specifically designed to uncover hidden biases and potential failure modes.
- Designing for Human-in-the-Loop (HITL): For high-stakes decisions (e.g., medical diagnoses, large financial transactions, critical infrastructure control, final hiring decisions), ensure that AI provides recommendations or analysis, but a human makes the final judgment or has the power to intervene and override.
- Prioritizing Transparency and Explainability: Where feasible, favor AI models whose decision-making processes can be understood and explained (interpretable AI). When using “black box” models, invest in techniques to approximate explanations and rigorously test inputs and outputs to infer behavior. Document decision processes thoroughly.
- Ensuring Robust Testing and Validation: Go beyond basic performance metrics. Test AI systems rigorously under a wide range of conditions, including edge cases and adversarial scenarios. Validate performance across different demographic groups to ensure fairness.
- Strengthening Data Governance: Implement strict protocols for data collection, quality assurance, labeling, and privacy protection. Actively seek out and mitigate biases in training datasets. Ensure data usage complies with all relevant regulations.
- Fostering Training and Awareness: Educate employees at all levels, especially managers and those interacting with AI systems, about the potential risks (bias, errors, ethics) and their roles and responsibilities in responsible AI use and oversight.
Conclusion: Vigilance, Not Blind Faith, is Key to Harnessing AI
The transformative potential of artificial intelligence is real, but so are the risks associated with its negligent management. The allure of seamless automation and cost savings can easily blind organizations to the dangers lurking beneath the surface of seemingly autonomous systems. Bias amplification, costly operational errors, and significant ethical breaches are not theoretical possibilities; they are demonstrable consequences of inadequate human oversight.
Treating AI as a fully autonomous agent ready to be unleashed without guardrails is a profound misjudgment. It ignores the technology’s inherent limitations – its lack of common sense, ethical grounding, and true understanding – and underestimates the complexity of the real world in which it operates. The belief that hands-off AI management is progressive is a dangerous fallacy; it is, in fact, a dereliction of duty that exposes businesses to severe financial, legal, operational, and reputational harm.
The path forward requires a paradigm shift from blind faith in automation to active, informed vigilance. Robust AI governance, characterized by continuous monitoring, rigorous auditing, human-in-the-loop design, ethical frameworks, and clear accountability, is not optional—it is essential. By embracing responsible oversight, organizations can mitigate the hidden risks and truly harness the power of AI not just for efficiency, but for sustainable, ethical, and trustworthy innovation. The future belongs not to those who simply deploy AI, but to those who manage it wisely.